Voyado has patented an algorithm within the e-commerce sector, which has gained great results for our clients. The algorithm optimizes the top-seller function and increases sales whilst also removing manual labor. Mikael Hammar, Head of Research at Voyado, tells us about how they came up with the idea and how it works.

Mikael Hammar, Head of Research at Voyado.
What was the problem you wanted to solve with this solution?
We observed that a default ranking doesn’t work well for a plain top-seller function. Longstanding products that were hot a couple of months ago still appear at the top of the list yet attract few sales, whilst new hot products don’t even make it within the top 10 positions. So this isn’t conducive to optimizing sales. At Voyado, we know how to fix that particular problem. Tests have shown that for a typical bookstore in Sweden, for example, if you use purchase data from the last day or two, you will get a good bestseller list for the first 10 products. Beyond that, you really need more historical data.
So here we need to consider some aspects of the problem. What do we mean by a bestseller list? Isn’t it just the item that has sold the most? Well, what time frame are we considering? Is it the last hour? The last day? Week? Year? Century? The time frame you choose is very important, and it depends on the purpose of the bestseller list. We want it to maximize future sales. In fact, our desire is to find a suitable algorithm that predicts what products a consumer will buy, given that we know nothing about the individual. As a second objective, we want to show what is popular right now, what the current trends look like, etc. We do this of course, to convince the consumer to buy.
We needed to solve the problem for all possible product lists that our customers (the retailers) have. Let’s take a bookstore as an example. Say that we, in general, want to list the products according to their bestseller rank. We want the same type of ordering on the home page as category pages. There can be thousands of category pages in a well-assorted bookstore. Every such category would have needed its own set of parameters in order to make such a rank “efficient.” Obviously, we don’t want to do this manually, even though our customers asked for such a feature. Instead of providing them with a parameter that they could tweak for each category depending on the context, we produced an automatic solution for the problem.
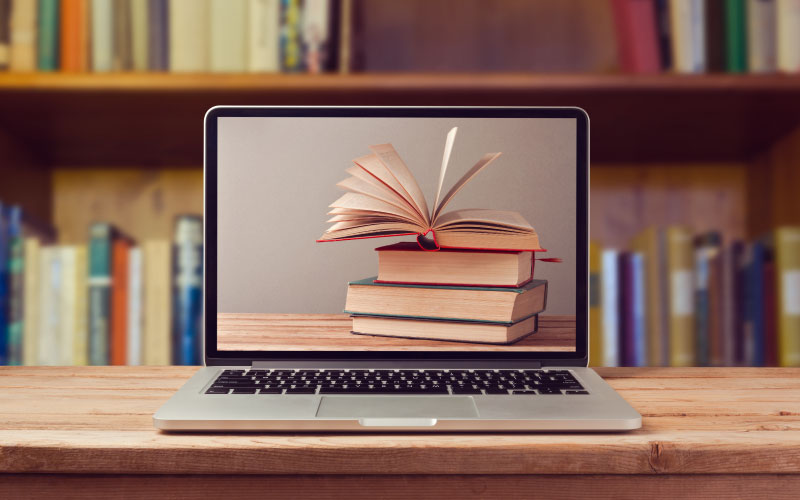
Can you describe how it works?
We decided that we wanted to order all products according to their probability of selling (or at least our best assessment regarding the sales probability).
To this end, we created a set of bestseller lists with different time frames, starting with a time frame of up to a couple of hours ago to a time frame of about two years ago. We made sure that the time frame selected covered the entire time span but did not have too many different groups. We ended up with about 10-20 different bestseller lists.
Next, we ordered the products in the following manner:
We started with the first position, call it X:
For position X, ask all bestseller lists to give us the best product for position X, given that position {1…X-1} is already known. That is, the products ahead in the list are already determined.
So each bestseller list will give us the candidate at the top of their individual lists that has not already been used in the resultant list.
From the choice from each of these bestseller lists, we choose the list that has previously shown to be the best at predicting the purchase at position X. This step is complex, and we need to make some approximations in order to select which list this is.
We continue this process until there are no more items to be ordered.

What makes it unique?
We can find a good sort order for any possible list of products right out-of-the-box. We do not need to tweak or learn any parameters manually, and we make sure that all products can be ranked. We can use the same algorithm for the bestseller list, for any product list, such as lists on category pages, and to sort the search results.
What effect have you been able to measure?
It is quite difficult to measure the effects on a site in a conclusive manner. At that time, we couldn’t do AB testing independently but had to rely on our customer’s own tests. So, the results are not validated.
Results from customers’ tests: The search refinements dropped by 50%. The conversion rate improved by 4.9%. It is about 10% better than manually curated lists.
The best result was that our customers were happy. They no longer get non-selling recommendations in their bestseller list, and trending items are captured swiftly by the list.
How can a customer take advantage of this within Elevate?
It is out-of-the-box in Elevate whenever you use the sort order “relevance.”